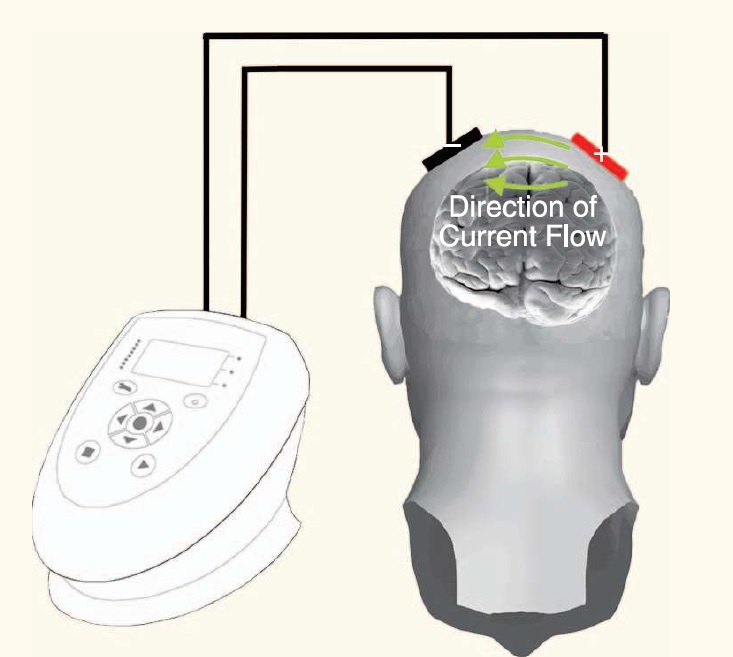
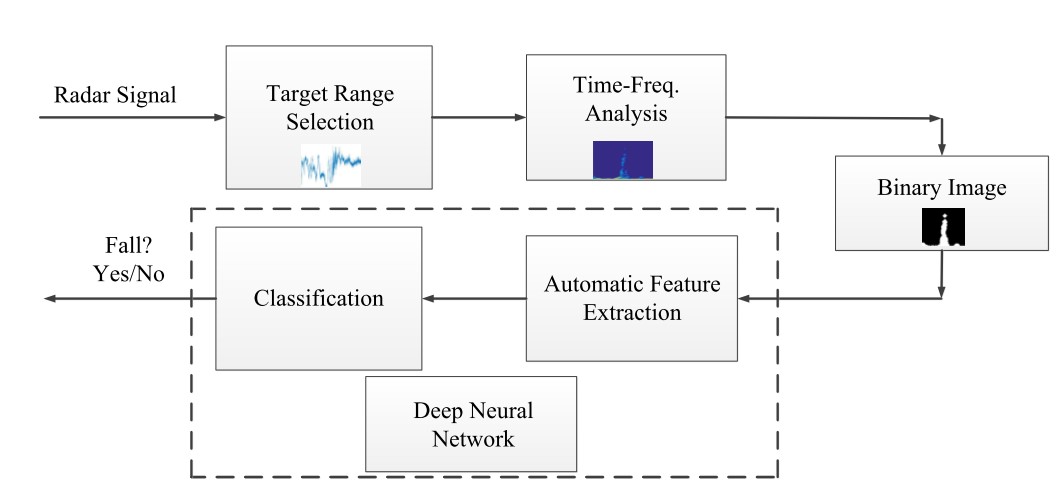
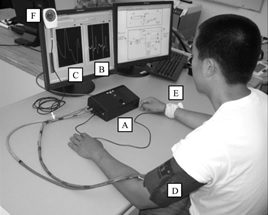
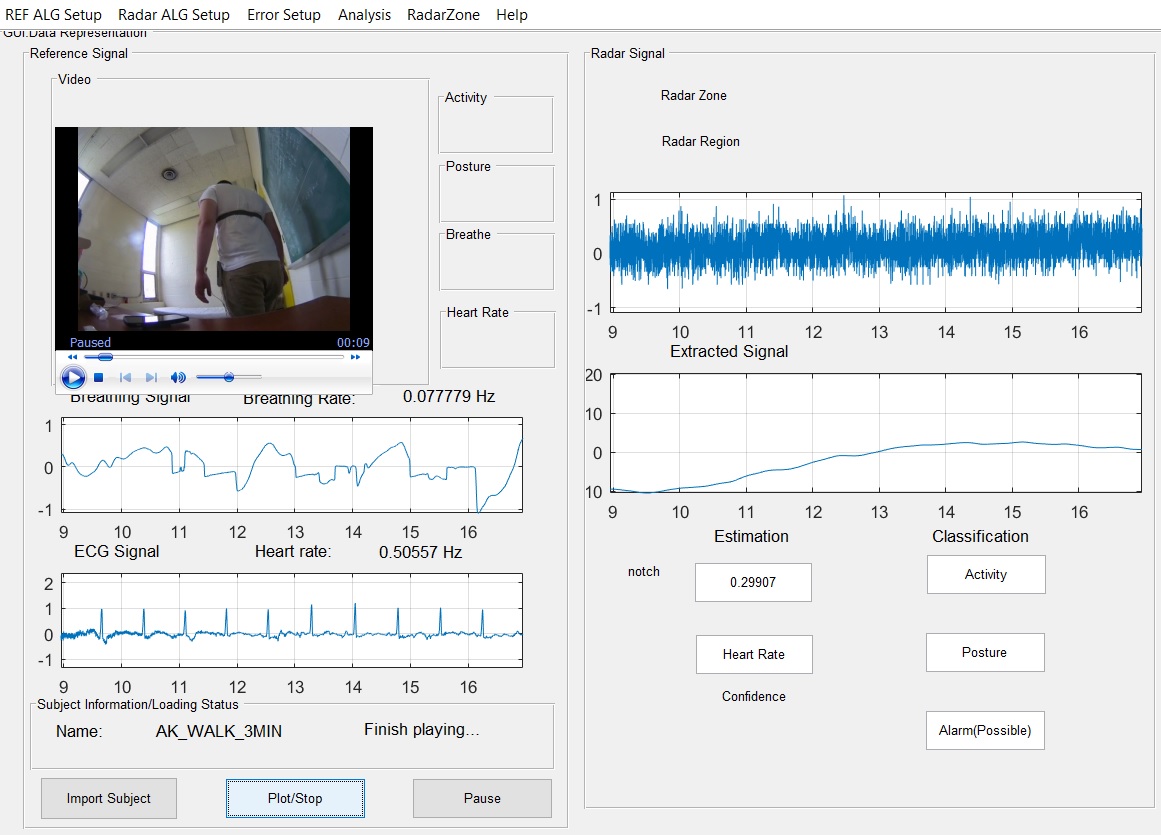
Current Projects
Monitoring elderly people in nursing homes
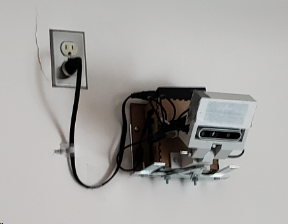
We will develop and test a contactless monitoring system intended for use by healthcare professionals for continuous monitoring of resident’s activity and breathing patterns as well as fall detection in assisted living facilities and retirement homes. The key benefit of our system is to allow for continuous monitoring without any impact on residents’ daily routines. Monitoring without any requirement for compliance by the residents or any additional burden to the nursing staff will allow us to collect a large number of data that will lead towards in-depth understanding of the activities and needs of the residents.
Description
-
The project is divided into two phases:
- Phase 1, which is the current phase, that involves data collection and offline processing for the purpose of validating developed algorithms. We will show the number of detected or missed events of interest (such as falls) as well the level of activities of the resident, the number of times he/she stands up in the night and so on.
- Phase 2, which involves deployment of the fully operating system that will monitor residents in real time and notify personal support workers in case of emergencies. This phase of the project is not going to be implemented at this time.
Members
Zixiong Han, M.Sc. student
Fan Yang, M.Sc. student
Dr. Miodrag Bolic
Industrial sponsor or collaborator
The Perley and Rideau Veterans' Health Centre
Status
Data collection has started in November 2019.
Thermal imaging for efficient detection of vital signs during COVID-19 pandemic
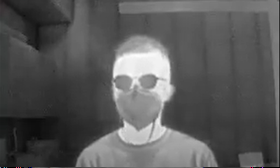
The goal of this project is to implement a stand-alone system with a thermal imaging camera that would allow for real-time processing and estimating the following vital signs of people: temperature, heart rate and breathing rate.
Members
Shan He, Ph.D. student
Fan Yang, M.Sc. student
Dr. Miodrag Bolic
Industrial sponsor or collaborator
Status
The project started in September 2020.
Classifying breathing patterns of heart failure patients
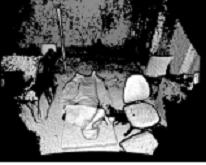
The objectives of the primary phase of this study are to develop and verify algorithms for estimating breathing patterns of each patient and for reliable prediction of decompensating congestive heart failure (CHF). By the end of this project, we will be able to demonstrate the ability of our technology to continuously monitor CHF patients. The key requirement is that the system be unobtrusive yet capable of continuous, accurate monitoring over distances of several meters. Initially, our prototype will be assessed in a hospital setting so as to corroborate data fidelity with currently available medical telemetry, now considered the gold standard. The eventual goal is to create a system capable of monitoring patients at home and relaying physiological alerts to care providers or emergency services in the community.
Description
This is a cohort study consisting of 10 patient cohort. The cohort will be studied prospectively by monitoring their breathing patterns with radar and 3D sensors. Breathing patterns will be manually labelled in the data, and algorithms will be developed to automatically identify the presence of abnormal breathing patterns such as Cheyne-Stokes or apnea episodes.
Members
Zixiong Han, M.Sc. student
Rajitha Hathurusinghe, M.Sc. student
Fan Yang, M.Sc. student
Dr. Miodrag Bolic
Dr. Peter Liu, the Chief Scientific Officer and Vice President of Research, Heart Institute
Industrial Sponsor/Collaborator
Status
Data collection has started in fall 2019.
Wearable devices for monitoring blood pressure
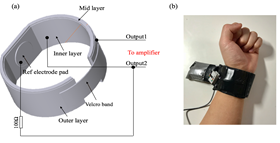
Smart watches in future will have smart wristband. This work analyses properties of new developed capacitive wristband sensor that measures ballistocardiogram (BCG) from a single point on the wrist. In addition, it considers applications of this sensor to monitoring heart rate variability. Another application is in estimating changes (trend) in systolic blood pressure continuously when combined with lead one electrocardiogram (ECG).
Description
Blood Pressure is one of the vital signs that indicates the health condition. It is commonly measured by cuff-based monitor using either auscultatory or oscillometric method. Cuff-based BP monitor is not portable and unable to measure BP continuously which means it is difficult to attach BP monitoring function on a wearable device. Significant research is conducted in estimating BP from pulse transit time (PTT) mathematically which would enable the cuffless BP measurement. In this work, a new time reference, RJ interval, which is the time delay between ECG and BCG signal peaks was tested whether it can be used as a surrogate of PTT in cuffless BP estimation. Based on the study done on 10 healthy people, it was shown that RJ intervals can be useful in evaluating trends of systolic blood pressure.
Members
Shan He, Ph.D. student
Dr. Miodrag Bolic
Coinvestigators
Dr. Hilmi Dajani
Dr. Glen Kenny
Status
Prototype of the new system has been made.
Published Papers and Reports
- S. He, H. R. Dajani, R. D. Meade, G. P. Kenny, M. Bolic, “Continuous Tracking of Changes in Systolic Blood Pressure using BCG and ECG,” EMBC 2019, pp. 6826-6829.
- S. He, X. Li, I. Batkin, H. Dajani, M. Bolic, “Detecting Cardiac Activity by Capacitive Electrodes from a Single Point on the Arm,” EMBC 2018.
AI and machine learning for development of insillico digital twin
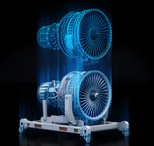
The long term goal of this project is to develop, test and make accessible to non-experts AI driven Digital twins for biological systems that can create and simulate behavior of biological molecules, network of interacting biological features from data providing a way to computationally design and test behavior of molecular and cellular systems in health and disease.
Description
The objective of this subproject is to develop ML algorithms and models that would allow for learning from heterogeneous sensor data as well as integrating traditional mathematical models with ML models. Although deep learning models allow us to model complex interactions and dynamics, they are difficult to interpret and require large labeled data sets for training. On the other hand, traditional mathematics models can be interpreted, but they are much simpler, which restricts their application. This work will allow us to benefit from the advantages of both types of models. We will develop probabilistic deep machine learning models that can include approximate dynamics of the system and utilize the traditional mathematical models and their parameters to constrain the deep learning model. In addition, the research in the field of probabilistic deep learning for time series is very new and models need to be improved and simplified to work in real-time as well as to be properly trained. The additional complexity represents the fact that we will deal with high dimensional heterogeneous sensor data from multiple sensors including oxygen input, carbon dioxide output, heating and cooling units, pH value, base addition, and agitation speed and relate these data to cell growth. Besides working on ML issues, we will also work on the software for the digital twin. The high level components/steps in designing digital twin include: building a virtual representation of the physical object, data collection, cleaning and analysis, integration of previously developed ML methods, validation of the model and uncertainty quantification.
Members
Dr. Nabil Belacel , Senior Research Officer within Data Science for Complex Systems Team of Digital Technology Research Center, NRC
Dr. Miodrag Bolic
Industrial sponsor or collaborator
National Research Council, Ottawa
Status
The project will start in April 2020.